Author: Professor Zhou Renjie, Guangyuan Zhao, Xin Shu, Department of Biomedical Engineering
As artificial intelligence (AI) transforms sectors from healthcare to industry, its substantial computation demand and energy consumption raise urgent sustainability concerns. Traditional AI relies on deep neural networks that require energy-intensive computations to process vast amounts of data. This approach demands significant computational power and substantial energy usage, posing environmental and economic challenges. A promising approach to addressing the high energy consumption of traditional electronic computing involves utilizing optical computing. This method employs light instead of electricity for computations, closely mirroring the structure of biological neural networks. Optical systems naturally support unidirectional data processing by manipulating elements such as light phase and intensity. However, these systems often face challenges when it comes to conducting training directly on the physical hardware.
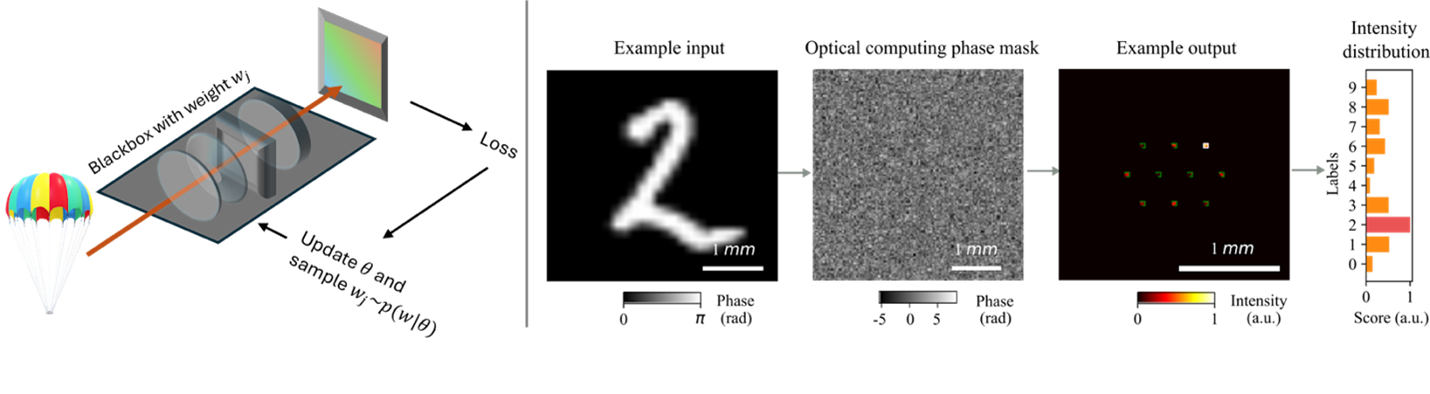 Left: The proposed method treats the optical computing system as a black box and back-propagates the loss directly to the optical computing weights' probability distributions, circumventing the need for computationally heavy and biased system simulations. Right: Visualization of experimental input and output of the trained optical computing system. The area with the highest brightness corresponds to the label of the digit ‘2’.
PhD Candidate Guangyuan Zhao, Postdoc. Xin Shu, and Prof. Renjie Zhou from the Biomedical Engineering Department have addressed this training issue by developing an innovative training strategy for optical computing systems. Their work, described in the ICCP&TPAMI’24 award-winning paper "High-performance real-world optical computing trained by in situ gradient-based model-free optimization," introduces a method that leverages a gradient-based model-free optimization (G-MFO) method, employing Monte Carlo gradient estimation techniques to efficiently train optical computing systems directly with real-world evaluations, bypassing the previous need for extensive and error-prone in silico computational simulations. This method treats the optical computing system as a "black box," optimizing it in situ—directly within its operating environment and utilizing light-speed evaluation—without the traditional reliance on physically based, resource-heavy, and erroneous simulations. By doing so, it significantly reduces computation overhead, energy consumption, and the simulation-to-reality (sim2real) gap typically associated with training physical AI systems.
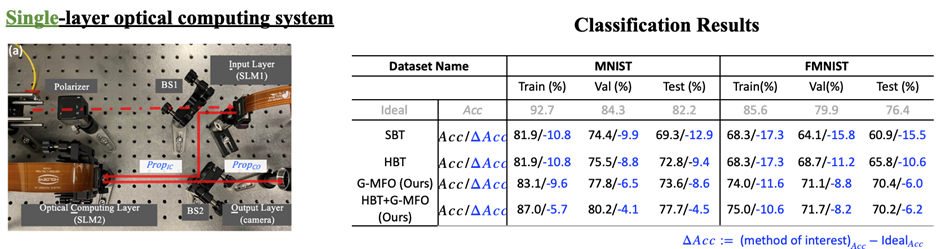
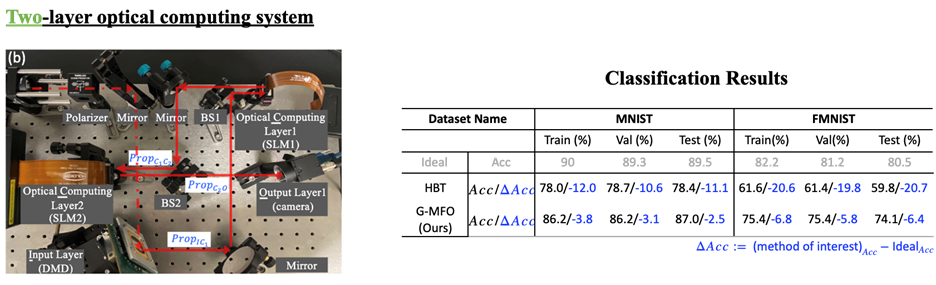 Left: The proposed method has been tested on the single- and two-layer optical computing systems. Right: Classification results on the MNIST and FMNIST datasets show the proposed methodology has resulted lower sim2real gap (as represented by absolute value of decreased Acc).
The researchers demonstrated the effectiveness of this system through complex tasks such as image classification and real-time phase map analysis of cells, which are critical in medical diagnostics and research. The system not only showed better mitigation of the sim2real gap over traditional solutions but also showcased its capability in applications where speed, accuracy, and efficiency are paramount.
This novel training strategy serves as a major step that expedites optical computing towards sustainable and cost-effective AI. Moreover, the success in optimizing the complicated optical computing system also unveils the potential of applying model-free optimization in other computational optics tasks, such as camera ISP and holography display, which are important for downstream applications in commercial photography, robotics, and AR/VR.
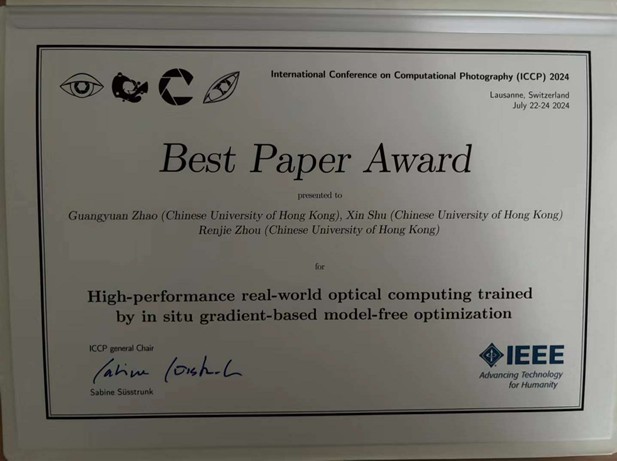
The paper for this research has received the best paper award at the International Conference on Computational Photography (ICCP) 2024.
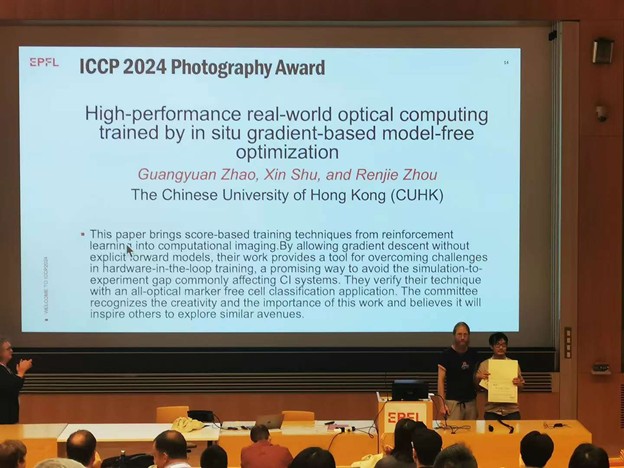 Comments from the ICCP 2024 best paper award committee.
The paper was awarded the best paper award and presented orally at the International Conference on Computational Photography (ICCP) 2024 in EPFL, Switzerland, in July 2024. ICCP is a well-recognized conference on computational photography and one best paper award is selected to recognize its outstanding research contributions. Mr. Zhao and Dr. Shu equally contributed to this work, under the supervision of Prof. Zhou. The paper is simultaneously accepted to the prestigious AI journal IEEE Transactions on Pattern Analysis and Machine Intelligence (TPAMI). Other teams participating in this conference were from Massachusetts Institute of Technology, Stanford University, Carnegie Mellon University, ETH, EPFL, Technion, etc.
For more detailed insights into this research, the full paper and additional information can be accessed on the project website.
|