AIoT Lab led by Professor Xing Guoliang won multiple awards at the 30th Annual International Conference on Mobile Computing and Networking (MobiCom 2024)
The AIoT Lab led by Prof. Xing Guoliang, has won multiple awards at the 30th Annual International Conference on Mobile Computing and Networking (MobiCom 2024).
Best Artifact Award
Title: “αLiDAR: An Adaptive High-Resolution Panoramic LiDAR System”
Jiahe Cui, Yuze He, Jianwei Niu, Zhenchao Ouyang, Guoliang Xing (CUHK, Beihang)
Best Artifact Award Runner-up
Title: “Soar: Design and Deployment of A Smart Roadside Infrastructure System for Autonomous Driving”
Shuyao Shi, Neiwen Ling, Zhehao Jiang, Xuan Huang, Yuze He, Xiaoguang Zhao, Bufang Yang, Chen Bian, Jingfei Xia, Zhenyu Yan, Raymond W. Yeung, Guoliang Xing (CUHK)
Best Runner-up Demo Award
Title: “Demo: 𝛼LiDAR: An Adaptive High-Resolution Panoramic LiDAR System”
Jiahe Cui, Yuze He, Yuchen Zhang, Jianwei Niu, Zhenchao Ouyang, and Guoliang Xing (CUHK)
ACM MobiCom 2024 is the thirtieth in a series of annual conferences sponsored by ACM SIGMOBILE dedicated to addressing the challenges in the areas of mobile computing and wireless and mobile networking.
PhD student Andes YL Kei and Professor Sherman SM Chow receive Distinguished Artifact Award at NDSS 2025
The paper “SHAFT: Secure, Handy, Accurate, and Fast Transformer Inference” by Andes Kei (IE PhD student and Hong Kong PhD Fellowship Scheme recipient) and his advisor, Prof. Sherman Chow, had received the Distinguished Artifact Award at the Network and Distributed System Security (NDSS) Symposium 2025. NDSS is a leading security conference. The artifact evaluation process, established in 2024, selected three awards from 68 submissions this year.
SHAFT is an efficient private inference framework for transformer, the foundational architecture of many state-of-the-art large language models. The team proposed cryptographic protocols that perform key transformer computations on encrypted data. SHAFT supports secure inference for large language models by seamlessly importing pretrained models from the popular Hugging Face transformer library via ONNX, an open standard for representing neural networks. This functionality provided by the artifact enables the machine learning community to deploy private inference on a wide range of models, even without specialized cryptographic expertise.
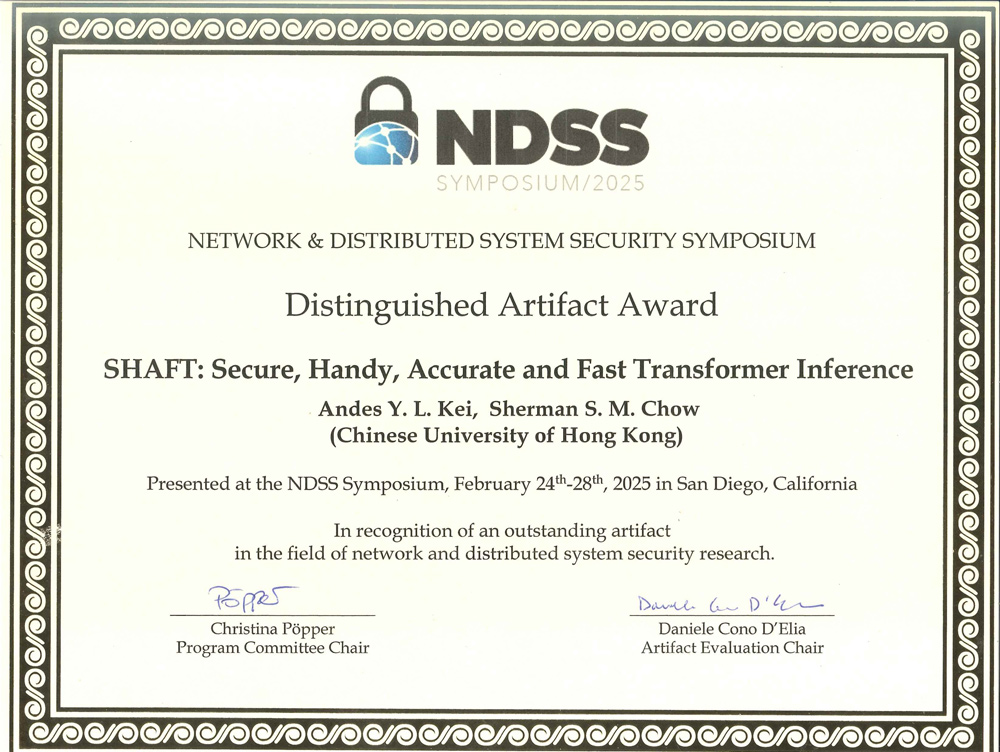
|