Imagine a future where robots naturally integrate into our daily lives, not merely following commands but genuinely understanding and engaging with their environment through sophisticated perception, manipulation, and embodied intelligence. This transformation is unfolding now as generative AI (GAI) technologies reshape the boundaries of robotics. From natural language processing to precise dexterous manipulation, from visual comprehension to touch sensing, from human movement demonstrations to coordinated robot locomotion and manipulation (loco-manipulation), these advances are creating robots that think, learn, move, and adapt in unprecedented ways, while developing an embodied understanding of their physical interactions with the world (Fig. 1).
  Fig. 1. Advanced robots for human
Key breakthroughs in generative AI are propelling this robotics revolution. Large Language Models (LLMs) enable natural conversation and complex reasoning, breaking complex tasks into manageable steps. Vision-language models like DALL-E and Stable Diffusion enhance scene understanding and response generation. Diffusion models transform robot perception and control by producing realistic sensory predictions and fluid motion paths.
By integrating these GAI technologies, robotics researchers are developing sophisticated capabilities for modern robots, especially humanoids. The combination of multi-modal foundation models for vision, language, and action with generative world models for simulation and planning allows robots to learn directly from human demonstrations, adapt to new scenarios, and engage in self-reflection through LLMs. This technological convergence enables robots to grasp complex tasks, learn from human behavior, and communicate naturally -- creating adaptable autonomous systems ready for real-world deployment. The MAE CLOVER lab demonstrates these advances through two examples below.
SayFuncGrasp [1] is an innovative system that enables robots to understand and execute natural language commands through LLMs. The system interprets the relationships between human instructions, grasp types, and object affordances, allowing dexterous robots to choose appropriate grasp poses. For example, when told to "Use the spray to clean a dish," the robot can select the right grip for the spray bottle, understand the task context, and execute the action effectively (Fig. 2). This advancement makes human-robot interaction more intuitive and accessible without specialized training, paving the way for versatile robotic assistants in homes and industry.
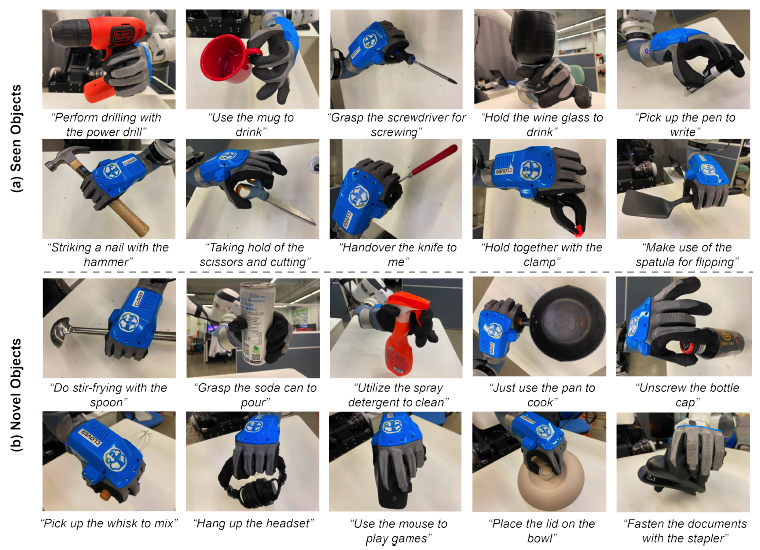
Fig. 2. Robot language-guided dexterous functional grasping
HOTU (Human-Humanoid Object-centric skill Transfer with Unified digital human) advances robotics by combining multiple GAI technologies to enable human-like robot behavior across platforms. The framework uses LLMs for natural communication and self-reflection, foundation models for visual understanding, and semantic 3D models with NeRFs and Gaussian splatting for environmental mapping. Its Unified Digital Human (UDH) model serves as a prototype for collecting human demonstrations, while adversarial imitation learning and model distillation optimize skill transfer for real-time robot operation. Through kinematic motion retargeting and human-object interaction mapping, HOTU breaks down complex humanoid control into functional components, enabling coordinated actions like simultaneous walking and object manipulation. This GAI integration has proven successful across multiple humanoid platforms, showing efficient skill transfer and reduced training needs while maintaining natural interactions (Fig. 3).

Fig. 3. Box carrying and putting task performed by unified digital human, NAVIAI and UnitreeH1 robots
Technologies, like SayFuncGrasp and HOTU, have transformed robotics by seamlessly incorporating GAI technologies across sectors, enabling robots to understand natural language and perform human-like actions through demonstration learning. By merging language processing, skill transfer, and motion control, these innovations have created versatile robots capable of complex tasks and lead to promising applications from precise warehouse operations to healthcare assistance with natural movements that reassure seniors, to household chores with unprecedented adaptability and safety.
CUHK, leveraging its world-class expertise in robotics and AI research, is poised to become a global leader in developing and deploying intuitive robotic systems. Through groundbreaking innovations, CUHK will revolutionize how robots operate in dynamic environments, such warehouses, health-care facilities and elderly homes, significantly advancing productivity, safety, and quality of life across multiple domains.
Note: The Collaborative and Versatile Robots laboratory (CLOVER Lab) led by Prof. Fei Chen from MAE, focuses on the co-evolutionary development of human-centered robotics and AI/GAI technologies for advanced robots, such as humanoid robots, to perform autonomous, assistive and collaborative tasks by learning and transferring the skills from humans.
[1] Fei Chen, et al., "Language-Guided Dexterous Functional Grasping by LLM Generated Grasp Functionality and Synergy for Humanoid Manipulation" in IEEE Transactions on Automation Science and Engineering, 2024 (accepted).
[2] Fei Chen, et al., "Human-Humanoid Robots Cross-Embodiment Behavior-Skill Transfer Using Decomposed Adversarial Learning from Demonstration" in IEEE Robotics & Automation Magazine, 2024 (accepted).
Author: Professor Chen Fei, Dapartment of Mechanical and Automation Engineering
|