Jia RuofanPhD in Statistics
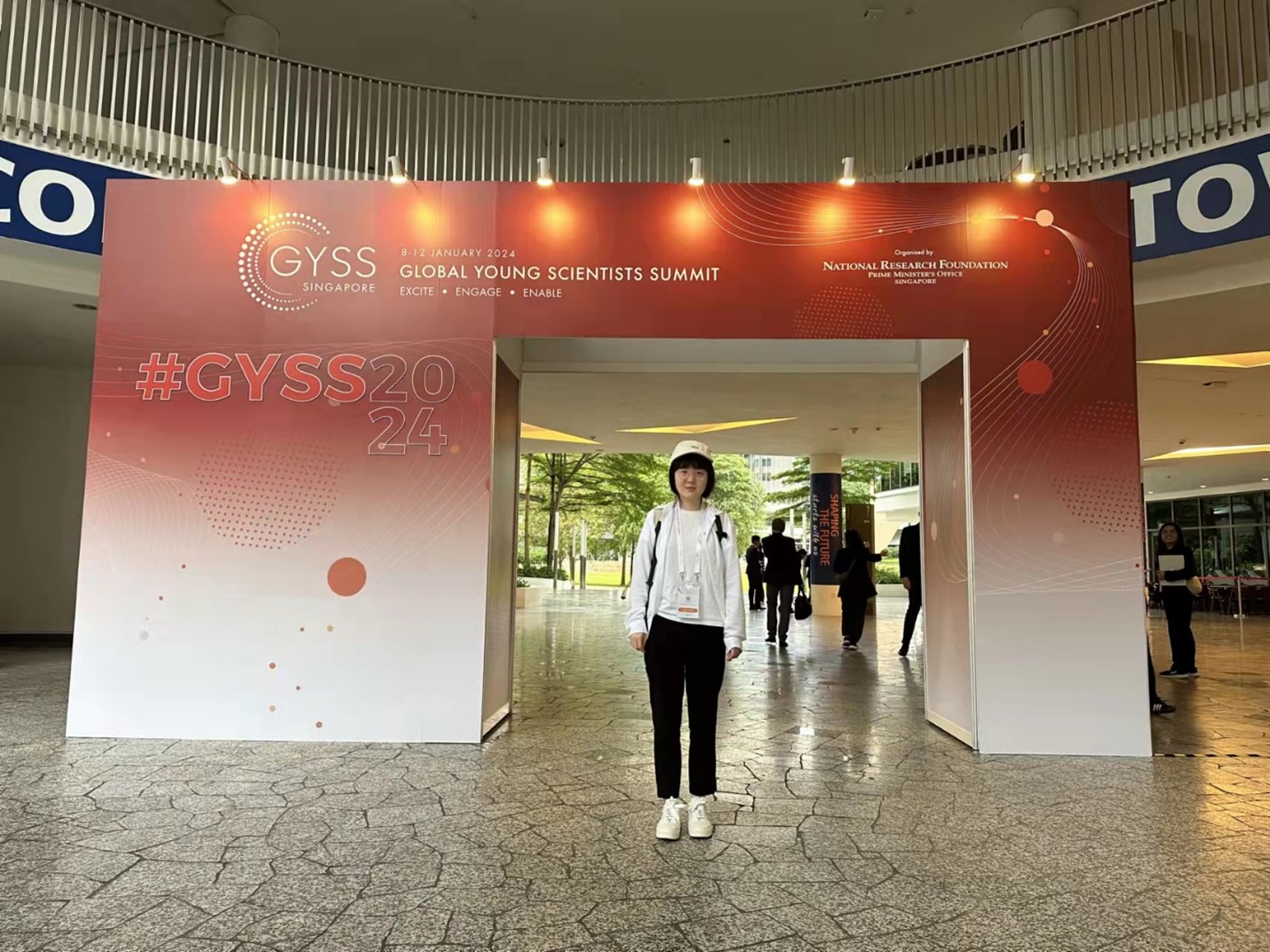
It was a great honour to attend the Global Young Scientist Summit (GYSS) 2024 from 8–12 January at the National University of Singapore. GYSS 2024 was a gathering of some of the top minds from all around the world and involved an exciting lineup of plenary lectures and panel discussions. Renowned researchers from different research areas shared their insights and pushed the boundaries of our understanding of science. It was also a fantastic platform for me and other young scientists to showcase our talents and exchange ideas via free networking in small group sessions.
One of the most mind-blowing lectures at GYSS 2024 was given by Prof. Adi Shamir on his new theory of adversarial examples in machine learning. Prof. Shamir won the Turing Award in 2002 and his expertise and contributions to the field are highly regarded. He introduced his recent cutting-edge work, which offers numerous insights into explaining the mystery of adversarial examples. These are perplexing phenomena discovered in 2013 that illustrate the extreme vulnerability of deep neural networks to small changes in their inputs and for which a straightforward explanation or means of testing has been lacking. Prof. Shamir explained adversarial examples in detail and introduced the dimpled manifold model, a novel conceptual framework that explains the evolution of the decision boundary between classes during training. In this model, the training process is divided into two distinct phases: a typically quick clinging phase, where the decision boundary aligns closely with the low-dimensional image manifold containing all of the training examples, and a typically slow dimpling phase, which creates shallow bulges in the decision boundary, positioning it correctly relative to the training examples. This framework provides a simple explanation for the existence of adversarial examples. Furthermore, Prof. Shamir revealed that a network trained adversarially with incorrectly labelled images may nevertheless correctly classify most test images and emphasised that adversarial training primarily deepens the dimples in the decision boundary.
I am grateful for being granted this valuable opportunity by the GYSS 2024 Organising Committee to be inspired by impressive and world-class scientists. I also highly appreciate the nomination from and support of the Department of Statistics.